Data merging is combining data from two or more sources into a single file. The best practices for data merging vary depending on the type of data being merged, the size of the data sets, and the desired outcome of the merge. Merging data can be a complex process, but it is often necessary to get a complete view of the data. By consolidating data from multiple sources, organizations can better understand their data and make better decisions based on that data. Keep reading to learn more about data merging best practices.
What Are the Best Practices for Data Merging?
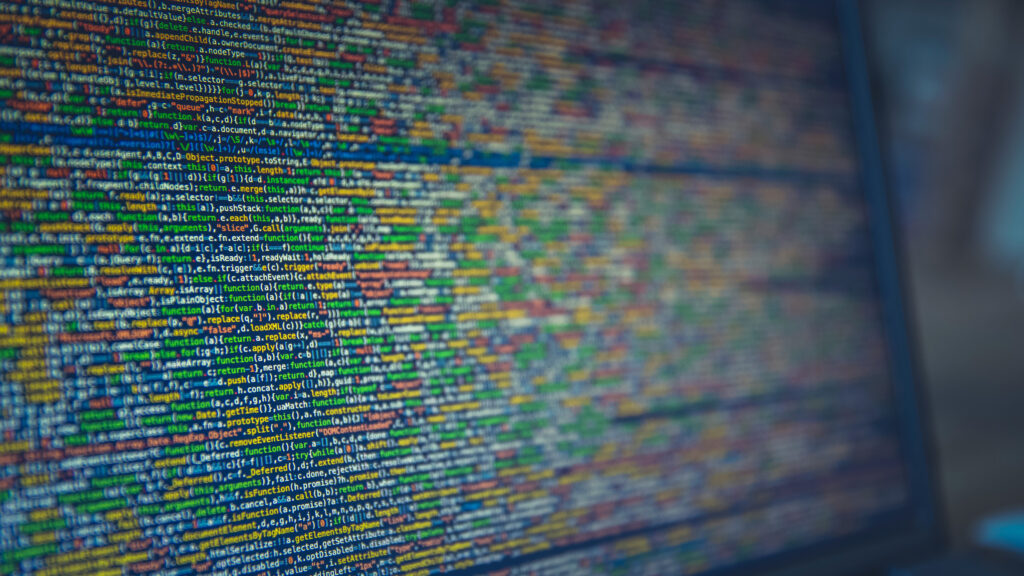
Merging data combines data from two or more sources into a unified data set. The goal of merging data is to create a single, accurate view of all the data to make better decisions. Several best practices can help you achieve this goal.
Before you begin any data integration project, it’s essential to establish a clear business need. What problem are you trying to solve? What decision do you need better information to make? Understanding the business need will help you focus your efforts and ensure that the result is something that provides value to your organization.
Once you know what problem you’re trying to solve, it’s essential to define the scope and requirements of the project upfront. This will help ensure that everyone involved understands what needs to be done and how it should be done. It will also help keep costs and timelines under control.
Use an appropriate tool for the job. Not all tools are created equal when it comes to data integration projects. Make sure you use a tool suited for the specific task at hand, whether it’s extracting data from one source, consolidating multiple data sets, or cleansing and transforming data before loading it into a database or warehouse repository.
Plan and allow plenty of time for testing and debugging. Data integration projects can be complex and time-consuming, especially if they involve integrating data from multiple sources with different formats and structures. It’s essential to plan to have enough time for testing and debugging; otherwise, you risk putting your project at risk of delays.
One key factor in ensuring the successful completion of your data integration project is using high-quality test datasets during the testing phase. If your test datasets aren’t representative of actual production data, your integration will not work as expected when implemented in production.
The use of the best practices for data merging is essential to ensure accurate and consistent data across all systems. Inconsistent data can lead to errors and inaccuracies, impacting business processes and decision-making. By using the best practices, businesses can ensure that all data is accurate and up-to-date.
What Are the Benefits of Data Merging?
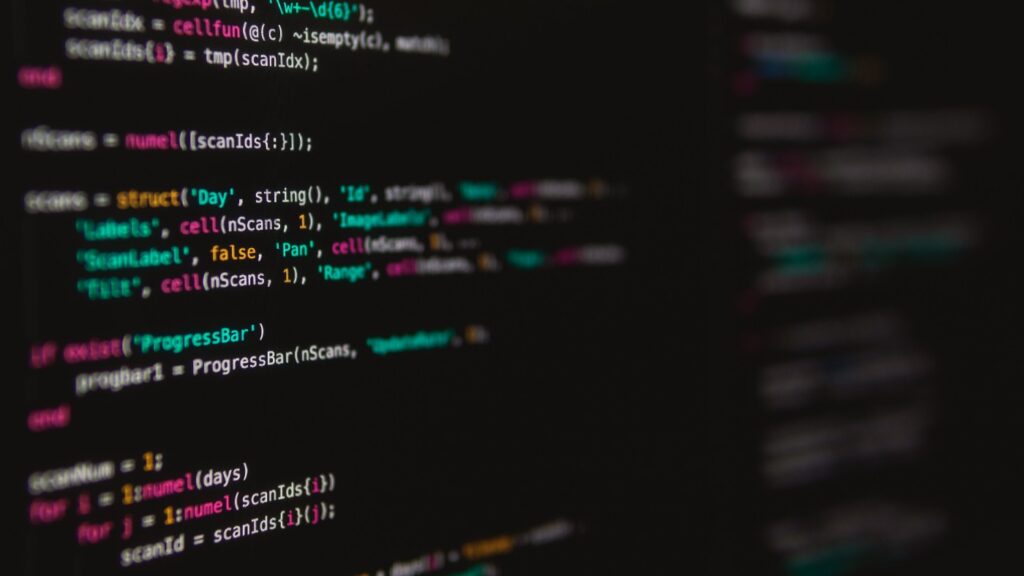
Data merging can combine data from different sources, such as various departments or business units within an organization or data from different periods. The benefits of data merging include:
- Improved accuracy and completeness of data: By combining multiple data sets into a single dataset, you can ensure that all information is accurate and complete. This can reduce the time and effort needed to clean up and verify the data.
- Increased insight and visibility into your data: Merging data can give you a more comprehensive view of your business operations and performance. This can help you identify trends and patterns that may not be visible when looking at individual datasets separately.
- Enhanced decision-making capabilities: The ability to see your data in its entirety can help you make better decisions about improving your business performance. You may be able to identify areas where sales are lagging or costs are high by comparing different datasets side-by-side.